The expansion of the market for security cameras equipped with AI features has led to a substantial increase in the volume of metadata transmitted from these devices. While this growth opens up valuable opportunities for enhancing the usability of stored video—such as enabling searches for specific objects (like people and vehicles) and their attributes (such as blue shirts or eyeglasses)— it also poses challenges regarding processing and storage requirements.
This article specifically addresses why the user may or may not want to store a particular type of AI metadata – the bounding boxes. Bounding boxes are visually seen as a rectangular shape around the perimeter of a specific object, such as person or vehicles, which has AI Classifications. They are stored as screen coordinates in the database and can consume significant amounts of available storage on the hardware that is used to run the exacqVision VMS.
Within exacqVision VMS, the setting to store bounding boxes is presented on the Event Linking configuration page of the Desktop client application. The retention time for bounding boxes is automatically defaulted at zero on new installations of ExacqVision VMS.
Note: The setting to store bounding boxes does not in any way impact the ability of the client to show them during live viewing of the camera stream
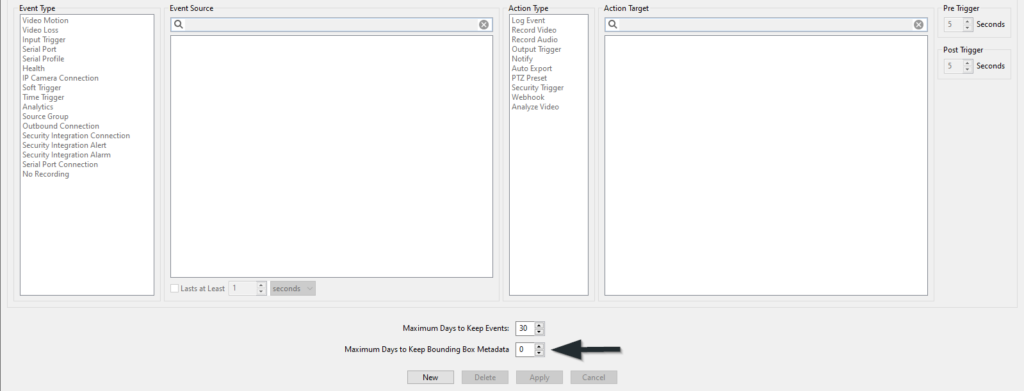
Factors to Consider When Deciding to Store Bounding Boxes
Enabling the disk recording of bounding boxes comes with the increased need for resources on the system. It impacts the storage rate of the system, including video storage rate. The system may also require increased RAM sizes to handle the load. This impacts adversely the number of cameras that can be connected to that specific system.
In terms of disk storage space, exacqVision currently uses ~100GB (approximately) for both alarm events, AI classifications and bounding boxes. Given that bounding boxes (and associated frame level data) are more than 95% of AI metadata that comes through, storing bounding boxes will lead to reduced retention time for alarm events and classification data such as people, vehicle, backpack etc.
That said, bounding boxes can be useful in certain scenarios
· An incident investigator is doing a forensic search looking for a specific object, say person wearing a red shirt. This type of filtering will still work without bounding boxes. That said, if the scenes on camera view is busy with various objects, say a parking lot with lots of activity, it can be useful to see bounding boxes with color bubbles at top of those bounding boxes. This helps to quickly pull the user attention to the target of the investigation
· For a security personnel involved in decision making around the choice of vendors and devices for their deployment, it is useful to evaluate various camera vendors for their AI capabilities. One of the metrics is the ability to identify different objects in the camera’s view as part of the search workflow. Bounding boxes help highlight this capability visually, thus providing a visible metric that can be used to compare the different camera offerings. Similarly, for a integrator or a sales representative doing a demonstration of the AI capabilities, having the bounding boxes show up during search provides that visual confirmation and builds excitement around the offering.
· When the system administrator or technical support is finding the solution is not functioning per expectations in the area of AI object classification – say the forensic search is not correctly finding a person wearing a red shirt. Bounding boxes can help in troubleshooting by making it obvious if the person and colors are getting classified correctly
Conclusion
Opting in to store bounding boxes – while it can reduce the number of cameras one can have on the system – can be useful in specific use cases such as those identified above. The decision to store them to disk should be taken after careful consideration on the trade offs .